Medtronic Internship 2024
U-Net Deep Learning Model for Arrhythmia Segmentation
Introduction and Background
During the summer of 2024, I interned with Medtronic’s AI Research Team within the Cardiac Rhythm Management (CRM) Research & Technology (R&T) sector. My work focused on training a U-Net deep learning model to segment episodes of a specific arrhythmia (an irregular heartbeat) under investigation by the team.
​
The arrhythmia in question serves as a critical risk indicator for more severe cardiac conditions, which can lead to sudden cardiac arrest and death. Accurate detection and segmentation of this arrhythmia within a patient’s electrocardiogram (ECG)—a data-driven representation of the heart’s electrical activity—are essential for effective patient monitoring and care.
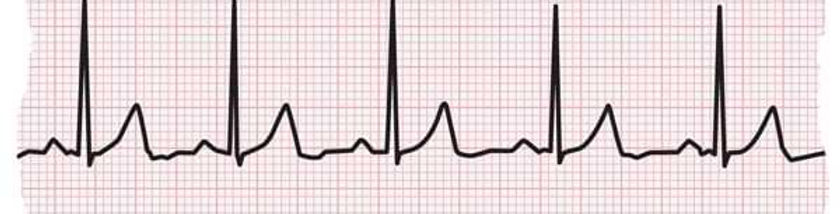
Figure 1: Example of an Electrocardiogram (ECG) of the Heart (American Heart Association).
Furthermore, with Medtronic’s LINQ™ Implantable Cardiac Monitor (ICM)—a small device designed to measure and collect patient ECG data—the team aimed to integrate the detection model into the CareLink™ system. This would enable the segmentation of arrhythmia episodes alongside existing AI algorithms.

The LINQ™ ICM is a compact, minimally invasive device implanted just beneath the skin of the chest. Positioned near the heart, it continuously monitors the patient’s electrocardiogram (ECG), providing long-term cardiac data. This small device is particularly valuable for detecting irregular heart rhythms and transmitting the data wirelessly to the CareLink™ system for real-time analysis and physician review.​
Figure 2: LINQ™ ICM (Medtronic).

Figure 3: Upper Chest Implantation Location of the LINQ™ ICM (Medtronic).
My Role
-
Identified and resolved annotation anomalies in patient ECG data using Python scripts.
-
Filtered and preprocessed ECG data, generating approximately 3,500 binary masks for model training.
-
Visualized ECG-mask combinations to ensure data quality and alignment.
-
​Trained a U-Net deep learning model in PyTorch Lightning, achieving a competitive mIoU score of 70%.
-
Presented project results and documentation to Medtronic’s CRM R&T Department and AI Research Team, showcasing its advantages over standard rate-based detection algorithms.
​
These efforts demonstrated the application of deep learning to improve the precision and reliability of arrhythmia detection in real-world medical applications.
Key Outcomes
-
​Trained a U-Net deep learning model with PyTorch Lightning, achieving strong performance metrics.
-
Set the groundwork for integrating the segmentation model into Medtronic’s LINQ™ ICM to enhance arrhythmia detection accuracy.
-
Presented findings to the Medtronic CRM’s R&T Department and AI Research Team.
-
Authored detailed project documentation for knowledge transfer and reproducibility.
Acknowledgements
I would like to thank my manager, Keara Berlin; my peer advisor, Robert Waxman; my peer mentor, Ryan Wyszynski; the AI Research team—Brandon Stoick, Andrew Radtke, Brett Jackson, Karissa King, Alex Famiano, Tarek Haddad, Sean Landman, Kelvin Mei, Adam Black, Maudeline Deus, Dillon Holm, Kaja Pederson, Wyatt Elmer, Michael Clayton, and Efia Barnes; as well as my fellow interns, Scott Li, Natasha Porwal, Ilana Andrev, Taylor Isabel, and Grace Condie. I deeply appreciate everyone I had the pleasure of meeting and collaborating with this summer!